Getting the most out of ChatGPT deep research
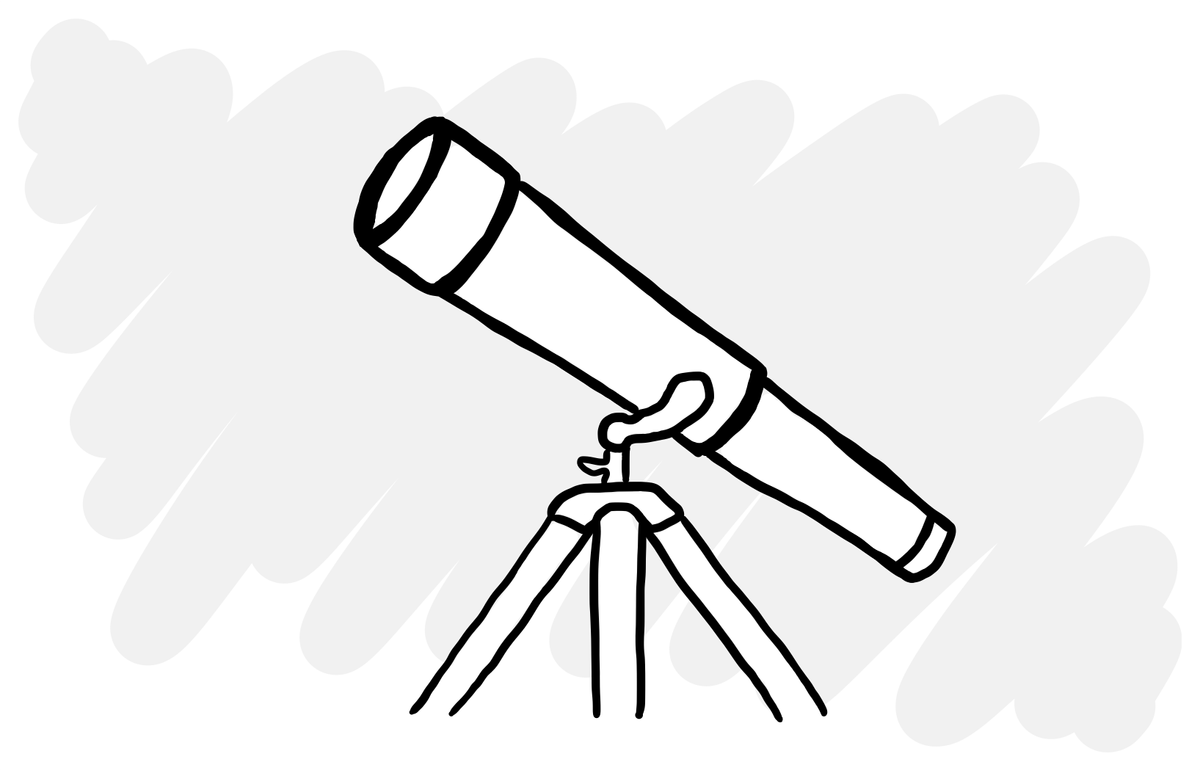
ChatGPT deep research has been out for a couple of months now. What is it good for and what are its limitations?
What is deep research?
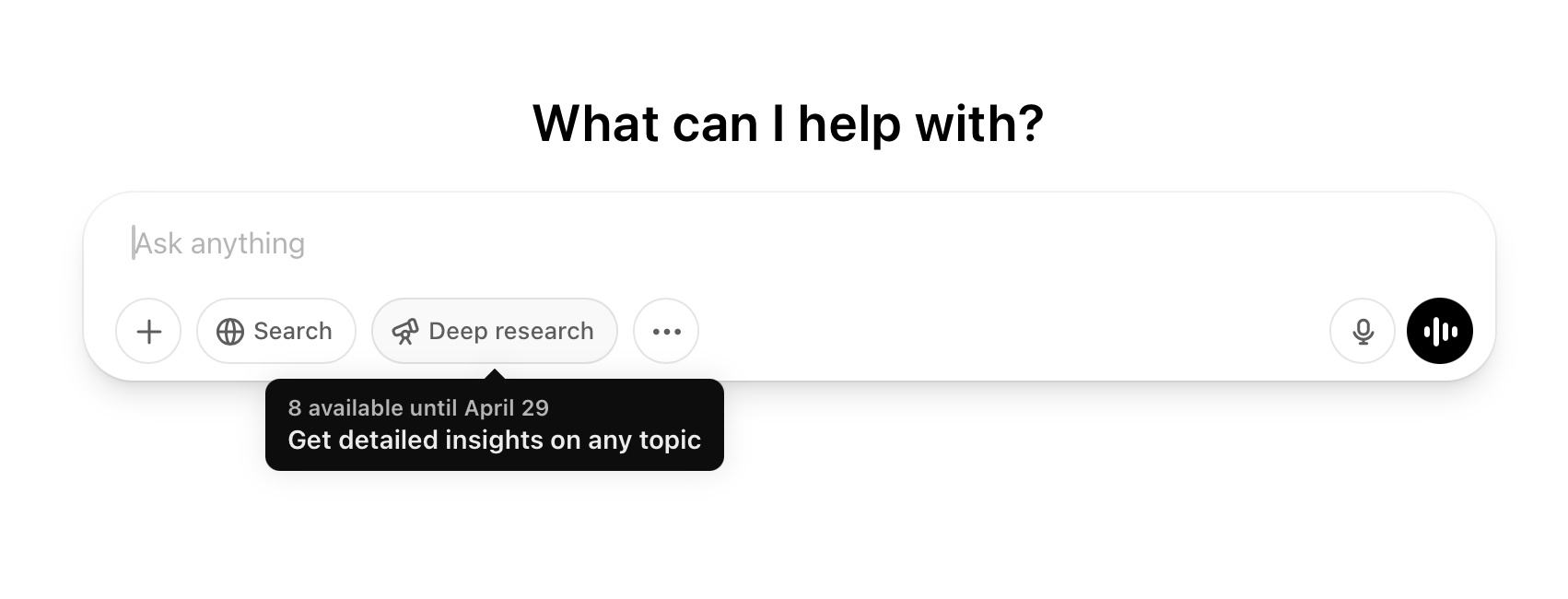
As the OpenAI website explains:
Deep research is OpenAI's next agent that can do work for you independently—you give it a prompt, and ChatGPT will find, analyze, and synthesize hundreds of online sources to create a comprehensive report at the level of a research analyst.
…
Deep research may take anywhere from 5 to 30 minutes to complete its work, taking the time needed to dive deep into the web. In the meantime, you can step away or work on other tasks—you’ll get a notification once the research is complete.
Essentially, the more time the AI has to complete a task, the better the results will be.
Deep research gives your prompt more compute, specifically to spend browsing the web and summarising what it finds. The catch is that you only get 10 queries per month on the $20/month Plus plan (or 120 per month on the $200 Pro plan!)
This makes it feel like a more specialist tool than anything else on ChatGPT - it’s more of a scalpel than a Swiss Army knife. It’s powerful, but narrower in scope, so getting good results depends on how well you write your prompt.
What it’s good for
- Niche topics where you know the information is out there, but it’s time-consuming to find, collate or analyse.
- Example use case (not the full prompt): Make a list of 30 newsletters in the areas of product, leadership, design and user research. Check the dates that the last three issues were published, then analyse this data to determine the most common day of the week that newsletters are published.
- Very specific queries with multiple parameters, and a standard LLM would not have enough time to work through the task.
- Example use case: Compile a shortlist of 10 rear-mounted bike racks that would fit a 26 inch wheel on a Nihola Family tricycle. I need to mount a battery on top of it, so it needs to be flat and at least 37cm long. It should be for sale in the UK at a price lower than £100.
- Summarising dozens of sources or multiple viewpoints.
- Example use case: What have Marty Cagan, Teresa Torres and other product management thought leaders written about the role and value of dedicated user researchers? What does the UX research community think about their viewpoints? Given the democratisation of research and the push for non-specialists to conduct research, what is the future role of dedicated UX researchers?
- Learning about a new topic in detail.
- Example use case: I want to understand the modern digital experience stack used by enterprise-grade websites and apps. Please break it down into categories and explain the role of each category in delivering, measuring, and optimising the digital customer experience.
Limitations
- You only get 10 queries a month (on the Plus plan), so you can’t use trial-and-error quite as easily to get to the results you need. If it doesn’t give you what you want, you need to put ‘another coin in the machine’.
- There’s a long feedback loop because it’s slower, so you can be waiting 20 minutes before you realise that it didn’t understand what you wanted.
- It always asks clarifying questions before it begins its task, but once it’s started then there’s no mid-task course correction.
- It’s only as good as what’s on the public web. If the data it needs is behind a paywall, in a book, in a YouTube video transcript, on Facebook, etc. then it will struggle.
- Because of the above, the quality of sources may vary, depending on the domain.
- It magnifies the problems of the standard ChatGPT, i.e. vague prompting and poor sources will lead to disappointing results
Tips for getting the most out of it
As with anything, the more you use it, the better you get at it. Here’s what I’ve learned so far:
- The longer and more prescriptive the prompt, the better. As Dare Obasanjo observed, “LLMs like ChatGPT reward detailed prompts with better responses, while Google search tends to degrade with longer queries.” Specify the role it is playing, give it context, clear task instructions and expectations of the output.
- Outline the types and age of sources it should use. Deep research using sources that you think are not authoritative enough is usually the biggest reason for getting a disappointing result. Ask it to prioritise or only use sources that meet a certain criteria.
- These prompts take longer to write, so get ChatGPT to write the prompt for you. Use the voice dictation feature to tell it what you want and let it craft it. You will likely need to go through a couple of rounds of iteration before the prompt is ready, but it’s faster than writing it yourself.
- Test prompts with regular ChatGPT (especially the o3 model) before using deep research. If you don’t need a big report, o3’s response can often be enough. In a recent query, it took over 3 minutes to make 20 searches and review 128 sources, without using up my monthly deep research limit.
- Use an emoji like 🔭 to remember which are your deep research conversations so you can easily find them later.
Deep research isn’t for everything, but when you’re dealing with a complex, time-consuming task that requires real-world data from across the web, it’s a powerful tool to have. Just know that it rewards preparation. If you treat it less like a chat and more like a project brief, you’ll get much more out of your 10 queries a month.